In the wake of ChatGPT’s sudden rise to prominence and its subsequent popularity, the arrival of more powerful artificial intelligence (AI) and machine-learning (ML) tools has led to a growing concern regarding the impact of bias present in these technologies.
In an interview with Lex Fridman, Sam Altman, CEO of OpenAI and creator of ChatGPT, contends that “there will be no one version of GPT [or AI] that the world ever agrees is unbiased”.
And as AI tools become increasingly embedded in various industries, bias has become a critical topic of discussion for lawmakers. Some have gone as far as prohibiting the use of AI tools in certain sectors.
A good example of this is France’s ban on predictive litigation AI, which aims to stop the commercialisation of tools that would allow users to accurately map judicial decision-making patterns of behaviour and predict case outcomes. The updated law in Article 33 of the country’s Justice Reform Law states: “The identity data of magistrates and members of the registry may not be reused for the purpose or effect of evaluating, analysing, comparing or predicting their real or supposed professional practices.”
Process more data
Now, what is interesting here is that this type of predictive analysis has been done by lawyers and law firms for years. It is part and parcel of litigation practice. What is different is the application of a tool that can process more data in a more attuned and comprehensive fashion than human operators or other, more basic tools, could – and therefore arrive at a result that is unnervingly accurate.
This is a problem because it undermines confidence in the neutrality of the legal system. Imagine being a defendant in a case, knowing that you are not guilty, but an AI tool giving you an assessment that there is a near certainty of you being found guilty simply because your case will be heard by a certain judge or magistrate. So, in effect, your guilt or the lack of it become completely irrelevant. The question needs to be asked – what then precisely is justice?
As other industries are accelerating their use of these technologies the question of bias rears its head in connection to other vital aspects of our lives, such as health and money.
As AI tools become increasingly embedded in various industries, bias has become a critical topic of discussion for lawmakers. Some have gone as far as prohibiting the use of AI tools in certain sectors.
Pfizer, for example, begun using IBM Watson, a machine-learning system, to power its drug discovery efforts in oncology in 2016. The hope was that this would speed up discovery efforts and bring drugs to patients more quickly. While IBM Watson did not live up to expectations, the arrival of new, far more powerful AI tools has led to renewed excitement in the industry. The advances in genomic sequencing that led to the swift deployment of the Covid-19 vaccine made people realise how new tools could lead to near miraculous clinical outcomes.
Global investment banking companies such as Morgan Stanley are also seizing the opportunity to incorporate AI technology into their operations. Andy Saperstein, Head of Morgan Stanley Wealth Management, explains that the firm aims “to leverage OpenAI’s breakthrough technology into a competitive advantage in how our financial advisors can harness Morgan Stanley’s knowledge and insights in ways that were once never thought feasible”.
Inequitable outcomes
The promise of exciting innovation is definitely there, but the fact remains that all of these AI systems require immense volumes of data in order to function well. Whether it is patient data, clinical trial data or bank customer data, the humans behind the code can introduce biases into algorithms that guide AI and machine decision-making processes. And this, in turn, can lead to inequitable outcomes.
Biases can enter data sets in many ways, such as through:
- Sampling bias – When a data set is chosen in a way that doesn’t represent the whole population fairly.
- Confirmation bias – When data is interpreted in a way that aligns with preconceived beliefs, while disregarding or downplaying data that contradicts them.
- Historical bias – When the data used to train the system is old and doesn’t reflect what is happening now.
Altman emphasizes that a good representative data set is crucial to reducing bias, stating that “a lot of our work is building a great data set.” Data sets are the foundation upon which AI models are built, and their quality and diversity directly affect the potential for bias in the models.
Utilizing data
Let’s revisit the wealth management case for a moment. Imagine that a wealth management system is fed a data set that includes historical information on foreclosures in the United States, which studies have shown often affect minority communities in a more profoundly detrimental fashion. The present day AI decision making will be based on this data set, with the historical bias of this data set potentially serving to amplify the already present confirmation bias of a final decision maker.
Or perhaps, a patient data set that is primarily composed of patients of ethnic Chinese ancestry (as would obviously happen if the drug research was done in China). A drug designed on the basis of that data set would obviously suffer from sampling bias and, perhaps, might prove less effective for patients of different backgrounds. This is obviously a deep issue given the fact that no country in the world is truly ethnically uniform and most have sizeable minority populations deserving good medical treatment equivalent to that available to the majority.
“Well-developed and tested algorithms can bring a lot of improvements. But without appropriate checks, developers and users run a high risk of negatively impacting people’s lives.”
Michael O’Flaherty, Director, European Union Agency for Fundamental Rights
In cases like this the AI tool itself is not at fault. It is guilty only of utilising the data in order to reach an objective pre-defined by the human operator and based on the data provided to it by the same person or group of people.
In effect it could be argued that one of the things that the proliferation of these systems is doing is holding up a mirror to the society that we live in. And sometimes that is a very uncomfortable, but perhaps also a very useful or necessary, thing.
Ethical guidelines and frameworks
As AI is almost certainly set to have a far-reaching impact on society and individual lives, policymakers are keen to take responsibility for addressing the potential harm and discrimination these systems can either cause or amplify.
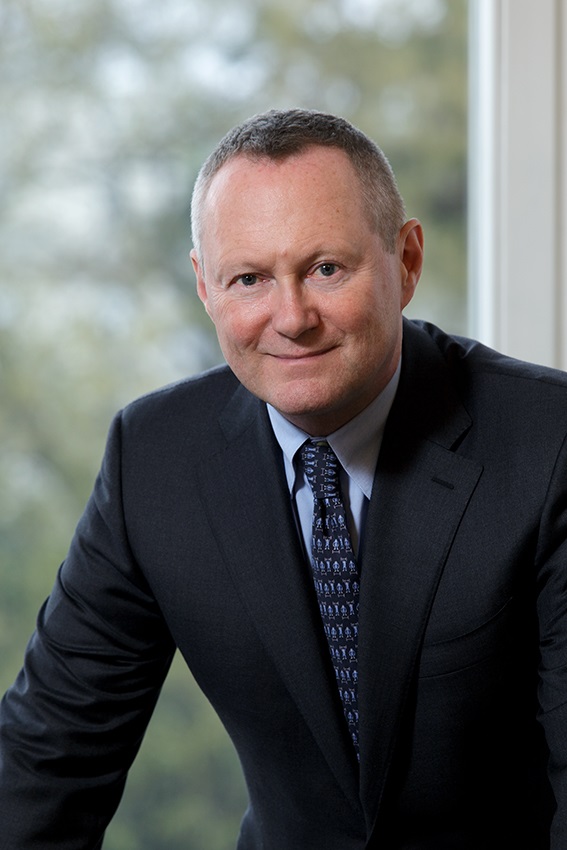
Michael O’Flaherty, Director of the European Union Agency for Fundamental Rights (FRA), expressed his concern about the potential risks, stating in a press release: “Well-developed and tested algorithms can bring a lot of improvements. But without appropriate checks, developers and users run a high risk of negatively impacting people’s lives.”
The European Commission’s proposed AI Liability Directive is a crucial move towards providing individuals harmed by AI systems with the same level of protection as those harmed by other technologies in the EU. This Directive is part of the Commission’s wider efforts to promote responsible AI development as part of its “A Europe Fit for the Digital Age” initiative, which aims to facilitate continuous digital innovation while offering clear guidelines and regulations to safeguard citizens.
While AI and ML offer many advantages, it is essential to minimize the presence of bias by developing ethical guidelines and required frameworks within legislation prior to their wider use in areas that affect people’s lives in a way that is more meaningful than asking a machine random questions. It is only with rules and frameworks that we can ensure that AI and ML continue to transform industries while promoting the key objectives of fairness and equality.
Aqsah Awan is an Analyst on Global Relay’s future leaders graduate program