Artificial intelligence is now in the mainstream. Its success is tempting many corporates to invest in research, experimentation and full deployment of a range of technology applications designed to optimize the performance of the enterprise.
Dr Matt Barrow leads the AI Lab at Global Relay. He entered the City trading environment in 1995 just as “rocket scientists” were all the rage. His Ph.D in Physics gave him the perfect profile for institutions such as Citibank, UBS and Credit Agricole where he built models and traded derivatives and structured products.
It was a natural progression from here to the world of data science, and many of his peers now combine their knowledge of the evolution of markets and financial institutions with the development of data-driven models such as machine learning.
Barrow has always had a strong personal interest in financial technology. After a spell as head of financial engineering for a company that sold pricing and risk models to investment banks and hedge funds, he dipped back into quantitative analytics and trading for a large energy trading company in 2010. “It was here that my early interest in AI was reignited. The data availability and increases in processing power began to make working AI solutions possible,” he says.
Financial technology
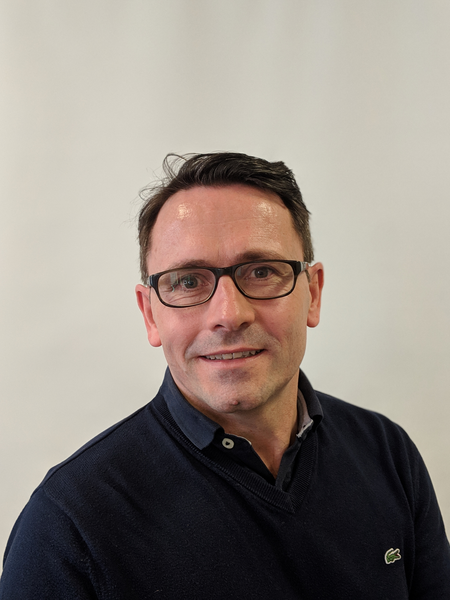
Photo: Global Relay
Barrow moved to Global Relay in 2019. He firmly believes that access to data is key and without it, no one can succeed. He also does not underplay the commitment required for the infrastructure and platform that supports this data, and emphasises the need for domain expertise to deliver understanding of use cases to help ensure the creation of fully optimized products. And he is candid about the scale of the challenge and the resolve needed to make it work.
“There has to be commitment and passion for experimenting with new models and technology and being aware that they might not work out as expected for the first time.
Without this philosophy, you will be trying to innovate within a culture of fear, you will get left behind in the AI space as it is moving so fast,” Dr Barrow warns.
“I have seen some very interesting compliance and conduct challenges in my 17 years on the front line of trading,” Barrow says. These include:
• mis-selling to a grandmother;
• outrageous gifts and entertainment abuses;• workplace bullying, violence and substance abuse
• covert employee monitoring;
• use of private detectives to monitor key employees deemed a flight risk;
• market rigging and collusion.
“Employees in the front office at financial services firms are highly incentivized to make as much money as possible for their institution and ultimately for themselves. As long as this incentive exists, there will always be compliance, conduct and operational risk,” he concludes.
Multi-Layer Perceptron
Matt’s first encounters with AI came in 1990 in an Advanced Neural Network class at University College London. He was introduced to the Multi-Layer Perceptron (MLP) – the most basic form of Deep Learning, which is an artificial intelligence function that replicates the workings of the human brain through data processing and pattern formation to assist decision making. Matt used this concept to build a basic Optical Character Recognition system that converts character images to text.
“It was very slow and not particularly accurate!” says Barrow, “then in graduate school I worked on image reconstruction and recognition projects – again using crude neural networks and advanced statistical deconvolution techniques – with a similar outcome!”
When he graduated in 1995, the obsession with neural networks was over. Early systems simply did not have enough training data and/or processing power to take advantage of the concept. It took 15 years for the trend to return, as big data and significant compute re-animated the field of AI like never before. Barrow was fascinated by the potential for market prediction.
“A financial market data time-series is a grid-like, labelled data set that lends itself to a whole range of AI models which when combined with auto-execution technology leads to where we are today with the majority of trades executed on major exchanges done by machines,” he says. The past 10 years have seen AI techniques percolate into many other areas and functions within financial institutions.
Tackle complex tasks
Sentiment models are ubiquitous, used to assess online shopping reviews, triage customer support queues, and much more. When used in conjunction with other models, such as language detectors, text classifiers, topic analyzers and traditional lexicons, these models can tackle more complex tasks such as identifying insider threats, reducing false positives in many applications, and modelling employee behavior.
The Covid environment saw a massive spike in electronic communication, through data platforms and systems that have dramatically grown the data sets used to train, hone and near-perfect the algorithms used to calculate sentiment, actions and behavior.
Simple models are now realistic for various types of action and behavior that are remarkably accurate – there is something in it for everyone.
“AI models are being deployed everywhere throughout modern organizations and across multiple functions. The key from a technology perspective is to understand what problems face each function. Then deploy the best model using stable, scalable and performant infrastructure that can be easily managed at a function and enterprise level,” says Barrow.
He goes on to describe the rather simple construct that helps deliver the intelligence required to form a powerful insight. AI algorithms obviously rely on source data such as text, audio, market data, and images. These algorithms produce enormous amounts of meta data. Imagine a smart doorbell which has a microphone and a camera.
An algorithm could produce the following meta data, says Barrow. “Recognized person as Matt. He is male, approximately 1.8m tall, Caucasian, about 25 years old, looks happy, wears Lacoste, waited 56 seconds, fidgeted while waiting, said (whispered) ‘answer the door I’m cold’, arrived in an Uber, left on foot.”
AI-related meta data
As another example, a corporation employs 100,000 people who on average send 200 emails and chats each per day. Each communication has on average 500 different pieces of AI-related meta data attached to it. These include attributes such as sentence-level sentiment, language used, the recipient of the message and so on.
Every day the organization is generating (100,000 x 200 x 500 = ) 10 billion pieces of meta data. All of this needs to be stored and rapidly retrieved to provide insight such as:
• who is an insider-threat today;
• why has this team been under-performing in the last six months;
• what groups of employees are talking about our latest deal;
• how can we decrease costs but still adhere to regulation.
This meta data is the source of actionable intelligence at an employee, group, business function and enterprise level. Barrow knows that there is a duty to widen this application beyond pure compliance, trading and regulated industry.
“I genuinely believe that companies like Global Relay have a unique opportunity to expand into many different business functions and domains. Electronic communication, whether through voice or text, documents or images, is used almost everywhere in every business of every size.”
“Understanding this communication and being able to act on its derived meta data will drive business value aggressively in the next 10 years. Soon a company will be at a distinct competitive disadvantage if it is not AI-enabled across all functions. It won’t understand its employees properly, won’t be able to service its customers properly, internal processes will be inefficient, operational risk will continue to be a problem, it won’t be able to plan finances properly. The list goes on and on.”
Barrow’s team has completed the formation of the AI Lab and AI Studio and a comprehensive selection of models. These are core pieces of technology that allow data scientists to securely build, train, test, deploy and manage models at scale.
“We are now in a position to respond very rapidly to our customers’ insatiable desire for AI solutions that solve a wide range of business problems. We take a very agile approach and we have to, because the AI space is so fluid and dynamic,” says Barrow.
“As we work predominantly in regulated industries, reporting is second nature. Making AI models explainable and auditable is part of our existing workflow and culture. Our simple model-layering approach is producing impressive results.”
Global Relay has created a big sandbox to play in, and is well positioned to deliver value across the functions of an enterprise. The goal is to extend the value to other departments within every enterprise such as IT, HR, Legal and the Sales teams. The outcome is intelligent information archiving where the living data allows stakeholders to really understand their business, their customers and their own talent.
Barrow is assertive with his view of the future in this area, “AI-enabled solutions are becoming so ubiquitous through modern organizations (and the products and services that they produce) that we are almost at a point where if a solution does not have AI components it is classed as legacy.
“It is becoming difficult to imagine a serious strategic foresight conversation amongst senior executives without AI being a major factor in any future vision.”