The report provides a great deal of detail on the current state of AI adoption by market participants including broker-dealers, asset managers, and exchanges. As such it represents a helpful industry benchmark and is a very useful read for those working in compliance as well as leadership and strategy functions.
Firms are increasingly using AI systems to support decision-making processes, but are also “considering using recent advancements” to support internal operations and processes including those connected with task automation, communications and risk management.
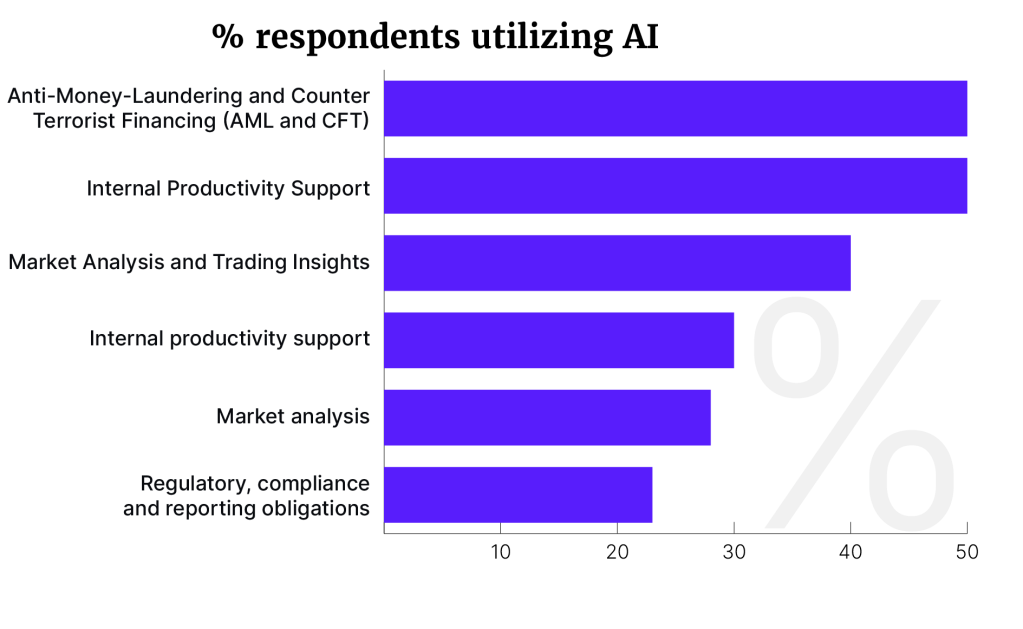
The risks most frequently cited by respondents included:
- malicious uses of AI;
- AI model and data considerations;
- concentration of outsourcing/third-party dependency; and
- interactions between operator/AI system.
The report present a landscape that is rapidly evolving with varying, but surprisingly high, levels of adoption across various functions.
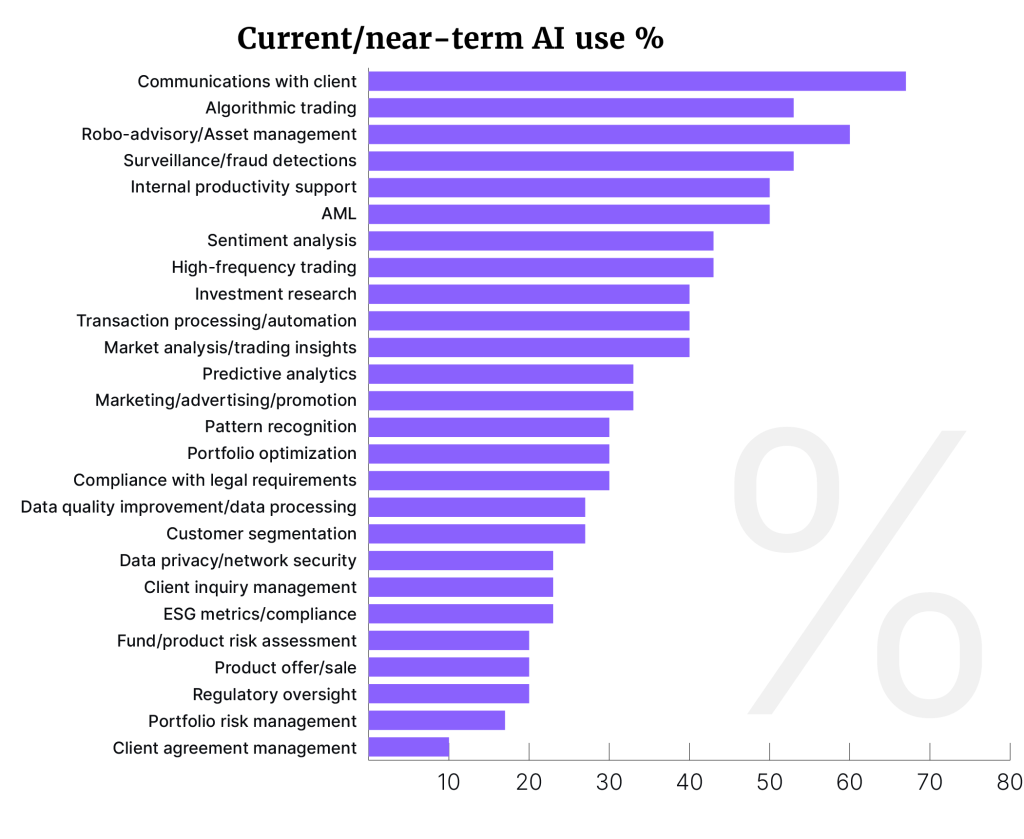
According to IOSCO the response by regulators is also evolving with “some regulators applying existing regulatory frameworks to AI activities, and others developing bespoke regulatory frameworks to address the unique challenges posed by AI.”
High-levels of AI use and adoption are being reported in connection with AML / CFT measures in particular with natural language processing (NLP) and machine learning (ML) widely adopted.
ML | NLP | |
---|---|---|
Anomaly Detection | x | |
Name screening | x | |
News analysis | x | |
Pattern recognition | x | |
Unstructured data interpretation | x |
Both technologies are employed to support the investigation process by:
- analyzing client behaviors;
- prioritizing red flags and suspicious activity; and
- integrating insights from other sources (such as news).
AI is also being used to bolster cybersecurity and risk management at firms, including in an effort to prevent and detect fraud.
In these applications AI is used to help:
- detect and analyze network traffic;
- prevent data leakage;
- segment customers by risk profile;
- prioritize alerts; and
- investigate specific activity.
When it comes to market analysis and trading insights AI is used most frequently to help firms with research as well as market and sentiment analysis. In this context the extraction and processing of information and insights from diverse data sources is one of the more common applications. But forecasting as well as trend and anomaly identification are also use cases reported by market participants.
The more recent advancements in AI (GenAI) are boosting the use of AI tools in order to improve internal productivity and operations at firms. Reporting adoption of AI tools are internal functions as diverse as software engineering and human resources.
An interesting development is the use of federated AI learning by firms to enhance surveillance measures with multiple firms contributing to the training of the model in order to improve detection rates.
Broker-dealers specifically report using AI for the purpose of communicating with clients, algorithmic trading and surveillance, and fraud detection.
% broker-dealers reporting use | Usage intel | |
---|---|---|
Communication with clients | 67 | Basic client query review and management with more complex matters escalated to human operator |
Algorithmic trading | 63 | Usage integrated across the trading lifecycle with latency being reported as a key challenge in algorithmic trading contexts where speed of execution is critical |
Surveillance and fraud detection | 53 | Potential to offer higher detection rates (than rule-based approaches) in the context of constantly evolving market behavior and manipulative practices |
Use of AI by asset-managers has a slightly different focus with AI most commonly employed in robo-advice / asset management along with investment research.
% asset managers reporting use | Usage intel | |
---|---|---|
Robo-advice / asset management | 60 | Supports automated investment advice and investment and portfolio management including optimization, customization and rebalancing of client portfolios. Also used in the identification of emerging investment themes. |
Investment research | 40 | Augments human decision making, helps with the monitoring of the macro-economic environment and facilitates analysis of foreign investment products as well as supporting client segmentation. |
Exchange and financial market intermediaries use AI most frequently in transaction processing and automation (40% of respondents). This includes both pre- and post-trade process automation and trade settlement.
The report includes a detailed and interesting section on risks stemming from the use of AI. Cybersecurity risk is a key concern here not only because of the potential of enhanced or automated attacks that utilize AI, but also because AI systems themselves can be vulnerable to attack. The report highlights the fact that because of their “technological features” attacks on AI systems can occur along the entire AI development and supply chain and could manipulate, training, output and potentially also exfiltrate information. But the use of AI can also “broaden attack surfaces” and “make cybersecurity procedures more challenging.”
There is real concern also about the fact that malicious uses can “lower the barriers to entry for bad actors” leading to more sophisticated ways of conducting fraud, cyberattacks and other misconduct. Mitigating techniques exist, but these are “not comprehensive and can be evaded.”
The report also tackles some of the risks associated with the models and data used to shape AI products.
Models
Issue | Usage risks |
---|---|
Explainability and complexity | Incompatibility with disclosure requirements. May lead to unsuitable recommendations to investors. Could inadvertently create conflicts of interest |
Limitations | Historical data sets used in training may degrade performance. Updates to commercially available models can unexpectedly vary behavior. Probabilistic rather than deterministic outputs can lead to diverging results. Fundamentally incorrect outputs generated in some instances |
Bias | Algorithmic bias in modelling. Cognitive bias in weighting and interpretation. Training data bias |
Data
Issue | Usage risks |
---|---|
Quality | Data utilized in training models can include inaccurate, imprecise, outdated, irrelevant or harmful content. Synthetic data generation can lead to fake or erroneous information entering into data sets resulting in data quality and reliability issues. Little transparency on what data has been used to train models |
Limitations | Insufficient sample size, which may lack information on events such as crises or “black swan” events, could undermine output reliability. Inclusion of irrelevant data or lack of data diversity can lead to “overfitting”, which means good results with training data, but not in response to real world inputs |
Bias | Overgeneralization and misinterpretation of data can perpetuate or amplify bias |
Concentration risk connected with outsourcing and third-party dependency is also a potential risk and a real concern for regulators. The small number of dominant tech providers means that concentration risk can arise across a number of different “dimensions” including:
- technological infrastructure;
- data aggregation; and
- model provision.
Market participants use open source and third-party AI applications to supplement any technology developed in-house. This has resulted in concerns about the regulatory perimeter, which currently does not extend to include technology providers. But as the role of the technology they supply to firms becomes more central to their operations they may need to extend to these in order to address concentration and resilience issues.
The report also addresses the talent gap emerging within industry in connection with AI tools and operations. There is concern that as a result of talent scarcity AI systems are inadequately understood and supervized, which may result in both investor and market harm. Over-reliance on technology is also an emerging issue and may result in the degradation of human skillsets over time. This, in turn, could lead to an inability by firms to supervise AI systems and ultimately lead to inadequate risk management by the firm.
Emerging risks connected with AI deployment include:
- elevated interconnectedness within the ecosystem;
- herding behavior that may lead to potentially significant increases in systemic risk; and
- coordinated or collusive behavior between AI models themselves.
Finally the report includes a very helpful section on the steps that market participants are taking to manage the risks identified including a section that specifically addresses third-party outsourcing considerations.